The Art of Communication in Data Science – through the lens of experience
Published by Subhadra Dutta | Assistant Vice President, Client Solutions
- January 4, 2022
- Posted by: admin
- Category: Data Science
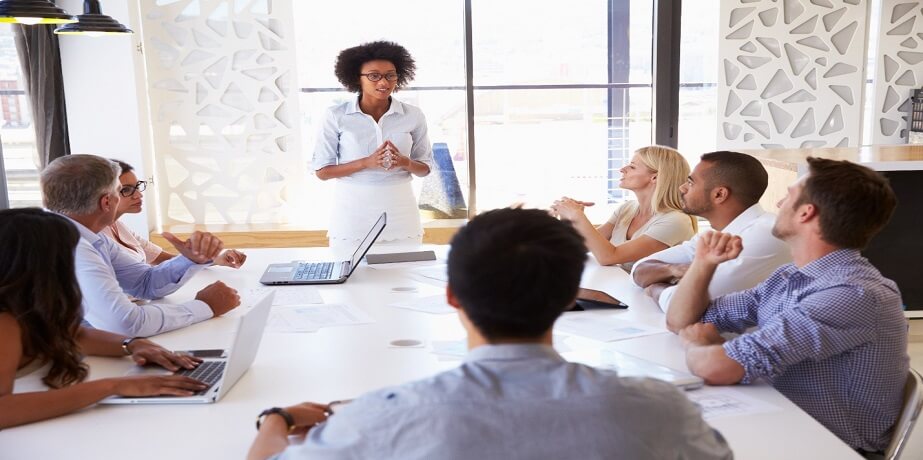
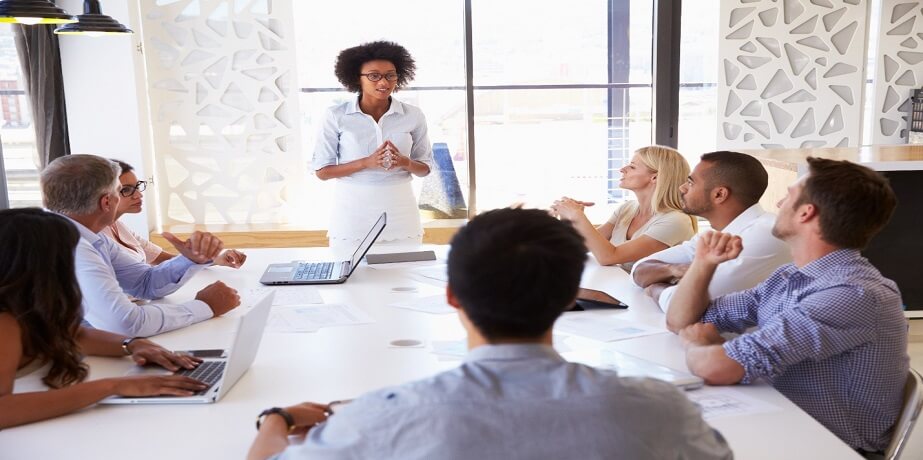
Communication is of paramount importance in every aspect of our lives. Data science is no exception. However, the additional complexity that Data Scientists live with is that they are required to closely interact with diverse groups of people even within a single project lifecycle. To put things into perspective, see the diagram below that captures the project lifecycle of a typical analytics project as per the internationally accepted CRISP-DM framework, and some examples of the various type of people involved at each stage.
In this blog, we at Analyttica Datalab want to provide you with some exposure around the different types of people you would work with at different stages of a typical project in data science. This diversified interaction requires customized communication across different people. We have compiled the finer nuances of communication across various types and seniority of people, from the vast and diversified experience in analytics consulting and delivery, The type and channel of communication would vary for different audience and so would the content. Here we will take you through a typical data science project lifecycle using the widely-renowned Seven C’s of effective communication – Completeness, Conciseness, Consideration, Clarity, Concreteness, Courtesy and Correctness. Depending on the type of people you are interacting with, both the order and the magnitude of each of these principles would change for most impact. Let’s understand this through the different stages of the project lifecycle of a typical data analytics project.
Stage 1: Business Understanding
This stage sets the basic context for the understanding of the business in general and the specific problem that you are trying to solve for. At this stage, you will be initiated into the project by the manager of the project at the client/business side. This person could be a product/marketing/analytics/business manager depending on the kind of organization structure the business has. This stage is foundational to the project and often to the relationship or contract as well, hence the communication should be capable of setting the right expectation with both sides. Clarity and concreteness would assume a big role within the communication principles here. Also, given that this stage is often the stepping stone for a new relationship, the degree of formality through courtesy and the ability to think from their point of view are also critical elements of the communication at this stage.
Stage 2: Data Understanding
This is the stage where a Data scientist gets into the actual business – data. At this stage, the interactions are mostly with highly technical individuals or teams such as Data warehousing teams, business intelligence teams or other data scientists. With these people, clarity, completeness, concreteness and correctness of the communication assumes primary role because the understanding of data of the client/business is the basic premise for any good analytical solution.
Stage 3: Data Preparation
This stage is similar to the previous stage in its communication ethos except this stage is an assimilative stage wherein, a lot of the learnings from the previous stage is processed and synthesized. This is also a stage where a significant share of communication will be with direct internal team members, hence the ability to leverage existing work relationships into communication will play a key role here.
Stage 4: Modeling
This stage, though called Modeling as per the CRISP-DM framework, actually refers to the creation of analytical solutions which would ultimately solve the business problem. This stage is where the data scientist often works with their own team to help create the solution. However, given that most of the work that goes into creation of this solution is highly technical, the communication needs to be articulated with clarity and conciseness. The ability to translate an analytical solution into a business solution is often the key differentiator between a good and great data scientist. This is also the stage where the recommendation of the data scientist is often the final word, as long as the client/business can interpret them. An analyst who has confidence in their recommendation as well as their analytical prowess usually does a better job in this stage.
Stage 5: Evaluation
This is the stage where the analytical solution – converted business solution is shared with the key stakeholder at the client/business side. This is where the data scientist shares their work with the work requestor and at times with senior team member in the client organization. This is where the art of storytelling in analytics plays out. The communication principles which are to be used in this stage should ultimately make your audience feel proud about the decision to engage you to work on their business problem. Hence, at this stage all the seven C’s of communication play a pivotal role. In addition, the way you lay out the content of the discussion, usually in a PowerPoint presentation becomes critical for this stage. There are many articles on the web providing various recommendations for presentation creation but one thumb rule which always helps is to try to think from a pyramidal perspective – the most senior person in the audience to the most junior person. What each of them would want to know for them to make a decision of evaluating your work for implementation, should be at the heart of your presentation content.
Stage 6: Deployment
This is the stage where it comes to life, and hence the most rewarding stage for a data scientist. At this stage, the analytical solution created by you would be implemented in the client/business systems and/or processes. Some examples of these are marketing campaigns, risk scorecard in marketing and collections systems, fraud detection rules in contact systems, proactive attrition flags in contact channels, recommendations in e-commerce pages, targeted advertisements on social media pages etc. At this stage, the data scientist would be interacting with various IT and execution teams to get their analytical solutions into the execution platforms of the client/business. Clarity, completeness, concreteness and correctness of the communication would become significant here to ensure that what gets implemented is what was recommended. What also follows the Deployment stage is the “Measurement” stage which is about measuring the impact of the solution from real-time implementation. This stage involves all the above project life stages and corresponding communication principles by turning the output into a story pre-and post implementation with concrete metrics, that can be understood in simple language. Ultimately this stage feeds into the Stage 1 as a continuous learning and feedback loop for future improvement.
Ultimately, communication is all about understanding and being understood. Hence at all times, the balance between listening and speaking needs to be maintained. As Einstein once said, “If you can’t explain it simply, you don’t understand it well enough.”