Gearing up for a career in Data Science? Don’t forget the Intangibles
Published by Subhadra Dutta | Assistant Vice President, Client Solutions
- January 4, 2022
- Posted by: admin
- Category: Data Science
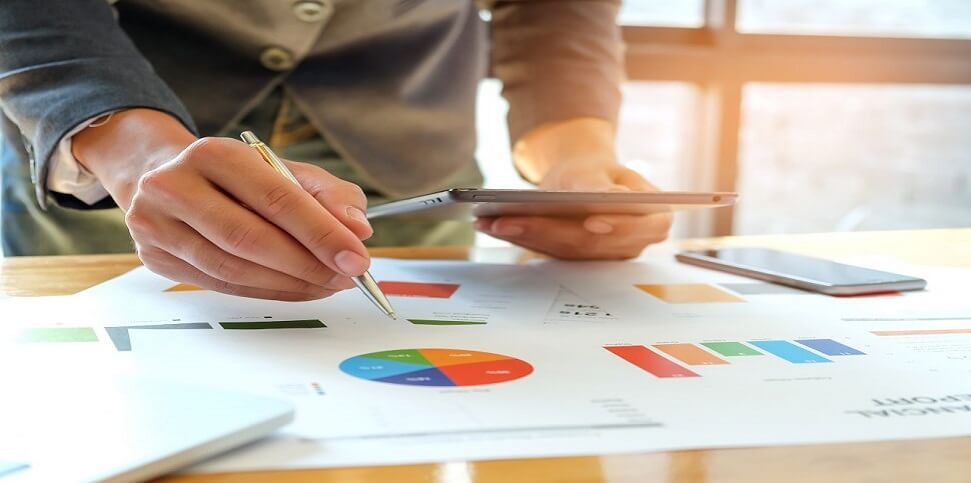
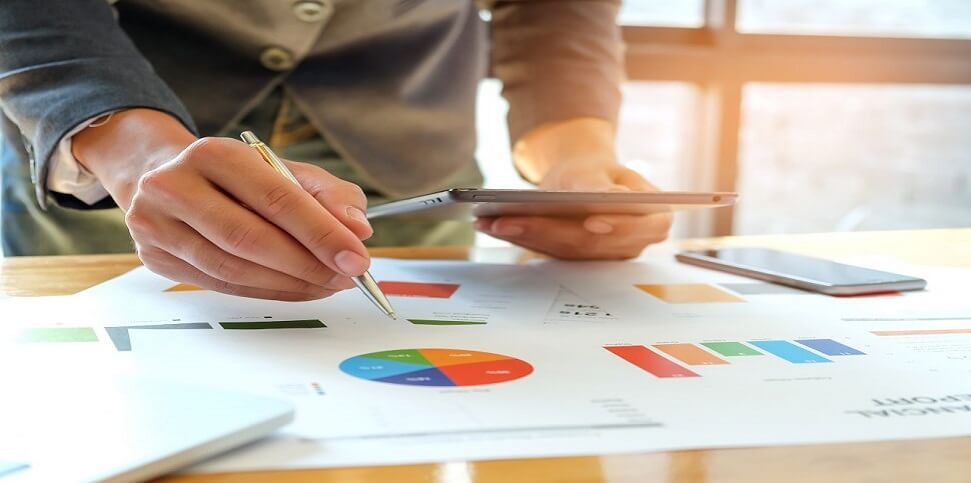
Are you wondering about the hype around Data Science? Data says that it’s not a hype anymore. Glassdoor released its Report of 50 Best jobs in America in 2017. With a job score of 4.8 out of 5, a job satisfaction score of 4.4 out of 5, and a median base salary of $110,000, Data Scientist jobs came in first for the second year in a row.
IBM predicts demand for Data Scientists will soar 28% by 2020.
Undoubtedly, a career in Data science is a lucrative one. Data science is an interdisciplinary field which can become a career option for most aspirants with appropriate training and self-motivation. Usually, the pre-requisite is an educational degree in any of the following disciplines — mathematics, computer science, economics, statistics, engineering, operations research or business management. However, aspirants from other educational streams can also get into this field with appropriate training. What is required is a dedicated focus on learning the hard skills that enable you to first get selected in a data science job and then perform and subsequently develop your career in data science. These hard skills include, but are not limited to, programming and data mining capabilities through languages like R, Python, SAS, SQL, Hive, Pig, Julia, SPSS etc as well as deep understanding of statistical techniques for data analysis and analytical solutions like basic descriptive statistics, hypothesis testing, regression and segmentation techniques and some exposure to advanced techniques like machine learning and optimization. The web is flooded with detailed information and guidance on these. Analyttica TreasureHunt could well-become a partner in this journey of yours, watch out for this platform.
In this race to get up-skilled and apply for positions that match the hard skill requirements, aspirants tend to overlook the “X-factors” that often turn out to be the deciding parameter for job selection. These factors, the Intangibles, are usually the unspoken qualities that employers look for in aspirants, when interviewing them for a data science role. The weight on these intangibles often increases with increasing years of experience. While you cannot gauge the exact questions around the intangibles during an interview, you can prepare for various situations beforehand and embed some of these into your day-to-day — this will help you become a stronger data science professional in due course. In this blog, we try to give you a snippet of five of the most important intangible skills, in an effort to kick-start your preparation for that job you have been eyeing in data science.
1. Investigative Mind
Curiosity and the hunger to ask questions fearlessly mostly make a great analyst . The ability to constantly investigate existing hypothesis and create new ones, often defines the key responsibility of many data science jobs. Data scientists are expected to spot changes in trends and identify the cause behind the change. This requires a strong eye for detail along with the tendency to associate causality with changes.
Employers adapt multiple ways to test an applicant’s level of strength on this aspect. It can be done through numeric, verbal and logical reasoning puzzles that you will be asked to solve during the interview. You can practice these through available resources online to be prepared for these. Interviewers tend to test the detail-orientation of the applicant through specific questions at each stage of the projects they have mentioned in their resume. These questions could range from your contribution, to the broader scope of the project. Interviewers will evaluate if you tend to focus only on the work that is assigned to you, or if you have a knack of understanding the business context as well. The best way to be prepared for this kind of discussion is to revise your earlier projects with a very structured approach in mind. And never, ever shy away from being honest to your interviewer regarding your involvement in the project.
2. Problem solving through Structured Thinking
Problem solving lies at the heart of Data science. It is the ability to break down broad business problems into smaller steps that often differentiates a great data scientist from an average one. The first step is to understand the business problem, convert that into an analytical problem, design the analytical solution and ultimately convert that into a business solution. The business problem must be broken down into certain hypotheses which then would be tested through the analytical process. The most critical component of that process is to seek all the relevant data sources and their accuracy. These hypotheses are tested using various analytical tools and techniques. Interviewers would give you a specific business problem and observe how you approach it. They will often ask you to create the various business hypotheses and question how you would validate those. The best way to be prepared for this part of the discussion is to practice various analytical problems as case studies with friends from the analytics or related fraternity.
3. Effective communication skills
Like many other jobs, effective communication is an important aspect of being a good data analyst. A great business analyst effectively trains the reflexes to think business first, and the analyses as an enabler, not an end to itself. That is how the analyses can be made to bridge the gap to the business metrics, and write/script a story on it in a way the stakeholders understand and implement. Communication skills for a data scientist role often includes but is not limited to verbal reasoning, proactive timeline management, making impactful presentations, negotiation skills, influencing skills and crisp email communication. Working in collaboration with the business teams and conveying complex analytical solutions in simple yet impactful business parlance is a crucial part of the job. The ability to interpret statistical outputs is a highly underrated skill in the analytics industry. Interviewers would test you on communication skills by asking you to communicate statistical results into simple language. The best way to be prepared for this part of the discussion is to understand the interpretation of statistical outputs in business language. The ability to translate the analytical outputs to simple language would be a differentiator in this part of the interview.
4. Lateral Thinking
One must develop the ability to apply existing methodology or solution to a completely new and different business problem. Here’s an example of survival analysis, which predicts the time until the occurrence of an event of interest, such as how long would a patient survive a certain chronic disease. This technique was used mostly in the healthcare and engineering industry. Now they are being used to solve business problems across industries. The interviewer would provide business situations from her industry and expect you to apply your learnings on that industry. The ability of leveraging analytical techniques from different industries is one of the primary reasons for organizations to invest in diversified data science talent.
The best preparation for this would be to become savvy on reading and learning from various resources — articles, blogs, books, videos, podcasts covering cross-industry application of analytical techniques.
5. Continuous Reinvention
Change is the only constant in today’s world, especially in this exploding pace of the analytics industry. The only way for anyone to survive and succeed is to constantly re-invent themselves. While traditional regression techniques could solve majority of the prediction problems few years back, Machine Learning and Artificial Intelligence are fast becoming the new mantra. While interviewers might not expect you to know these in-depth, unless specified in the role requirement, they would nonetheless expect you to have a point of view and broad understanding of these techniques — when do you apply them and what are some merits and demerits of the same? The best preparation would be to keep upskilling yourself through webinars, conferences and online courses covering a wide range of topics in the data science space. These self-motivated learnings definitely create a positive and lasting impact both on your potential employer and yourself as a future data scientist. The key to success is to remember the age-old philosophy of making your own mistakes and learning from them. Always be true to your real-self, you will have better grasp on any conversation by being truthful. Wish you a fulfilling career in data science!
P.S. Don’t miss how Analyttica TreasureHunt can become your partner in this journey to a successful career in data science! Watch this space for more.